Madrid R Users Group (RUG) is a community of industry professionals from a diverse range of backgrounds that provides a learning and networking platform. The organizing committee of the Madrid RUG recently engaged in a conversation with the R Consortium regarding the use of R in their respective industries. The organizers, Carlos Ortega, Pedro Concejero, Francisco Rodriguez, and José Luis Cañadas shared their valuable insights about the industry’s evolving landscape of R applications.

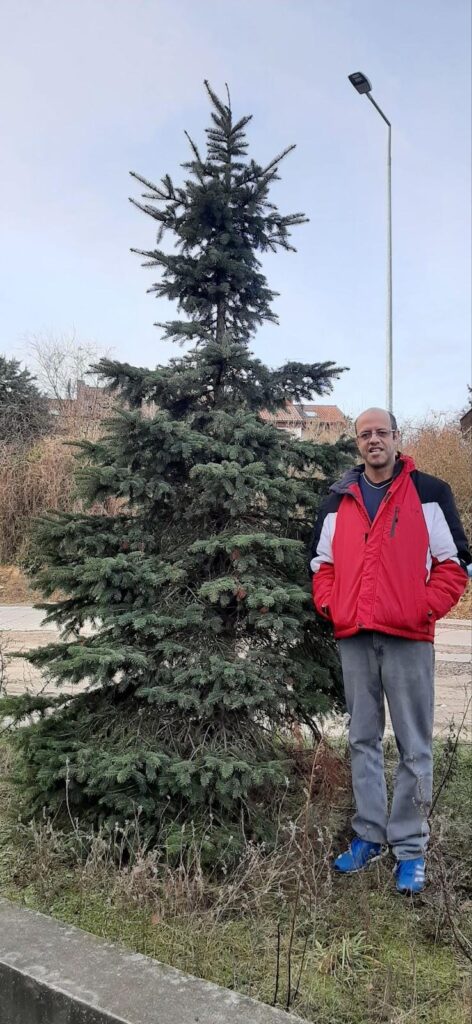
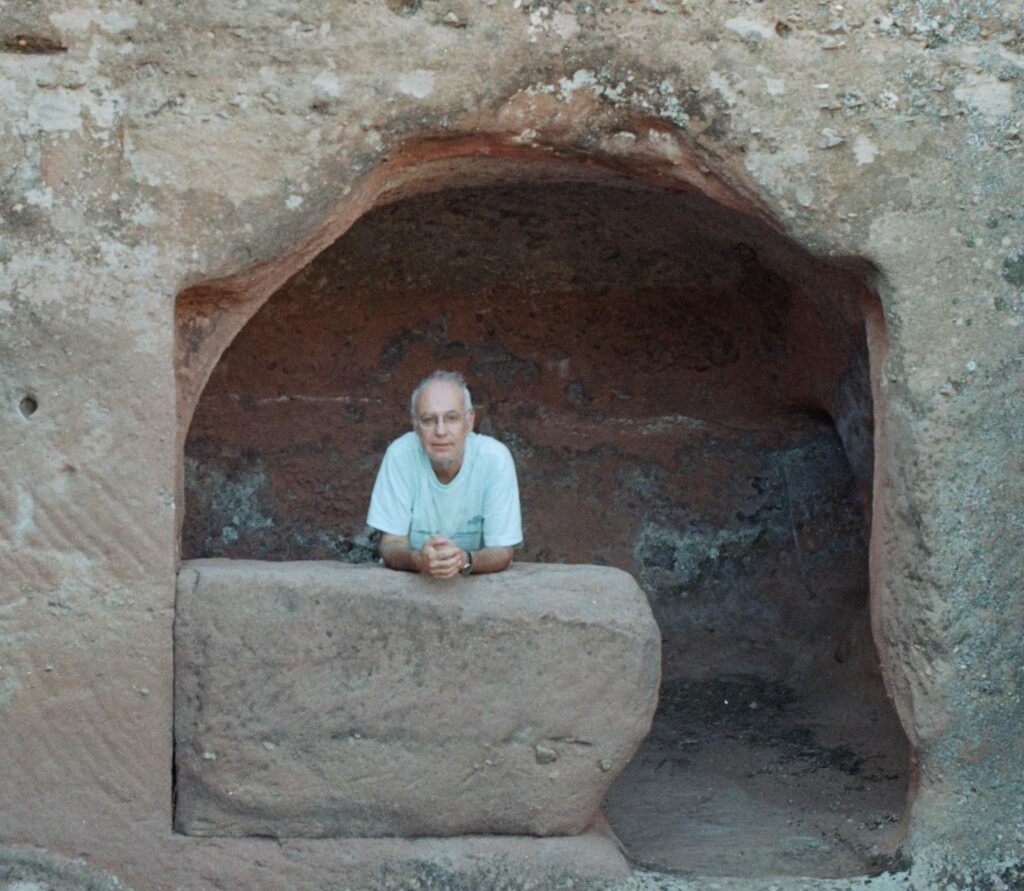

Please share about your background and involvement with the RUGS group.
Francisco: I began learning the R programming language in 1999 when I started my Ph.D. program. R was primarily used for command-line programming, but I did not use it seriously until 2012, 16 years later. Since then, I have used R in various enterprise settings, including insurance companies and banks. I have used R to process data and build models, and I continue to use it in my current role in the financial sector.
Carlos: I began my career at AT&T Microelectronics, a semiconductor plant in Madrid. We collaborated with Bell Labs, who invented R and used it heavily in the manufacturing plant. We collaborated with many colleagues, including John Chambers and his team. Some of their team members in the statistical group at Bell Labs laboratories were in our plant, sharing their code. When they started using R, we also started using it. However, the factory has since closed.
I transitioned to the consulting industry, working independently without formal affiliation to a particular sector. I analyzed data in various industries, including finance, manufacturing, and telecommunications. I worked in consulting for several years, and later with Francisco at a Spanish telecommunications company called Orange, and previously in the banking sector. We applied various models, analyses, and algorithms using distributed computing on servers and clusters.
I am currently employed in the service sector for a multinational human resources company. We place thousands of people into employment. Since the 1990s, I have used R to model, analyze, and clean data for many years.
Jose Luis: I am a statistician employed by Telco in Orange, Spain. I have been working in Orange for approximately five years. My primary job is to work with a Spark cluster using “sparklyr”, and the H2O library “sparkling.” I am a big fan of “sparklyr,” tidyverse, and dplyr. dplyr and sparklyr are the most useful tools for my work.
Pedro: My academic background is in psychometrics, and I have used nearly all the major statistics software, including SAS and BMDP. I came to R when I started working in data science for my previous company, Telefonica. In 2011, I began learning R on my own, and I enjoyed it immensely. I then met some people I am working with now when I started teaching psychometrics with R at the university. They thought I was crazy, but I enjoyed it, and it was a success. I then worked on many projects at Telefonica, including social network analysis with iGraph. I particularly enjoyed making prototypes with Shiny.When Shiny first appeared, it was a marvel, especially for those of us in data science who wanted to create systems, web pages, and prototypes. It was quite successful. I left Telefónica four years ago, and I miss it sometimes. Now I teach text mining and artificial intelligence at the university. I use Python for artificial intelligence, as I find it a bit easier. But I teach the rest of the machine learning and text mining with R.
Can you share what the R community is like in Madrid?
Carlos: As I mentioned before, we meet monthly. We work in different sectors: Francisco in insurance and finance, Jose Luis in telecommunications, me in services, and Pedro in education. Pedro previously worked in telecommunications as well. Most of the people we invite to our sessions are from industry. We invite a few people from academia from time to time, but most of our activities and recent developments are from industry. Therefore, industry is currently the focus of our group.
I joined this group because of its diversity, especially in the applied world. Many people are working on different projects, and their diverse ideas can be inspiring. In this sense, I believe that academics sometimes become too engrossed in their circles. The real-world applications of R are found by meeting people with diverse backgrounds.
Pedro: All of our meetings are recorded and uploaded on our website since 2010 or 2011. The credit for this goes entirely to Carlos who has consistently maintained this website.
Why do industry professionals come to your user group? What is the benefit for attending?
Carlos: The most important part of the meeting is the social events that take place afterward. The meeting itself typically lasts 40-45 minutes, during which we present new things, such as applying the Spark package or H2O library. Recently, Francisco presented how to apply a scorecard method for risk models in banking. Pedro presented how to use Shiny or Shiny mixed with GLMs or other types of models. Many people attend these meetings to see how effective R is in the real world. After the meetings, we discuss the state of the industry, such as which companies are betting on R and where the good projects are. This is a great way to socialize and discuss our issues.
Pedro: Now that Carlos has introduced the topic, I believe that the debate between Python and R is pointless. In my experience, both languages can be used seamlessly, and there is no difficulty in switching between them. My experience at Telefonica has shown that the choice of language can depend on the background of the project team, but ultimately, the results are the same. However, I must mention that the documentation available for R is excellent. I believe that R has a significant advantage over any other framework in the industry for statistical modeling.
The vignettes for packages are particularly helpful, as they provide detailed information on how to use the packages. Additionally, I believe that it is much easier and faster to start doing data science in R than in Python. Python is chaotic, with different versions of the language and packages being released frequently. R is more homogenous, with backward compatibility being a priority for the R Foundation. This makes it much easier to maintain a consistent environment in R.
While Python may be easier to use for some tasks, I believe that R is the better choice for professional data science work. It is more stable, has better documentation, and is more widely used in the industry.
Shiny in Python was released this year. However, when I teach about Shiny in Python, I tell my students to read the documentation in R. This is because the two languages are very similar, with only minor differences. For example, ggplot and Shiny are both available in both languages. As a result, students can simply copy and paste code from the R documentation and use it in Python. This will allow them to quickly and easily create powerful applications.
Francisco: The process of creating training, connecting to a database, extracting data, viewing the data loosely, creating a button, and distributing all the applications to all the people in your enterprise is relatively straightforward. However, the security of the data must be taken into account. If the data is compliant with GDPR and can be shared, then it can be quickly made into an application that can be viewed on a mobile phone. This can be done in Python, but it is more difficult. I prefer to use R for training.
Jose Luis: The use of plumber or other API packages has made it easier than ever to deploy R models in production. Kubernetes and Docker have made this possible.
Carlos: The approach to production is changing significantly with PosIt (former RStudio). Rather than having packages isolated, the focus is shifting to how to produce models in the enterprise. This means bringing R and Python closer to a real environment. These packages are making things much easier.
Pedro: For example, when I teach machine learning, I often use Python. However, when I get to mixed models, which I know José Luis is interested in, I have to recommend that students install an R library. This is because no Python library for mixed models is as comprehensive or well-developed as the ones available in R. At least two years ago, this was the case. I believe that R is currently years ahead of Python environments for statistical analysis.
What trends do you currently see in R language and your industry? Any trends you see developing in the near future?
Francisco:In my sector, banking, I remember that 15 years ago, SAS totally dominated the modeling part. Before 2010, it was the best option. It was not only a language but also a solution that allowed you to create a core model easily without coding. However, it was very expensive. Nowadays, I have noticed that R methods have been appearing in the industry since around 2015 or 2016. For example, in 2017, I was a consultant and taught a course to the regulator here in Spain. The Central European Bank wanted to use R to inspect bank entities, which was surprising to me, as I had not expected another country to use software other than SAS. Currently, we are testing R using a library created in 2020 called “scorecard,” which I believe is a powerful library.
This library, with only a few lines of code, enables building a complex model step-by-step and putting it into production easily. In my current job, I can use SAS or R. Here, given the choice, I use the easier and faster one, which is R. You can access data quickly with R, without any issues with the network. You use your computer’s memory, which gives you a lot of freedom to use the data you want.
I believe that the flexibility of this library in particular is causing many data scientists to transition to open-source. It includes people who use Python since Python has copied this library, “scorecard.” Formerly, one had to pay to use it. The quality of the model is comparable, if not better. What you are using here is a logistic regression.
Carlos: In the service sector, the use of dashboards has been on the rise. Power BI, in particular, has seen a significant increase in popularity, surpassing Tableau. QlikView has all but disappeared from the market. Now, the two leading dashboarding platforms are Power BI and Tableau. However, I believe that the industry is poised for a major change. R is a powerful tool, but it requires a machine with the R engine installed. Imagine being able to use R in a serverless mode, like in WebR, even through a web browser. This would revolutionize the industry by eliminating the need to pay for licenses. Dashboards could be published and shared with colleagues for free.
In essence, the way we expand will change significantly, as we will use R or Python to make it very easy to create and distribute dashboards at no cost. I believe this change, as well as the things that are working now at Posit, could have a major impact on our industry but especially in the service sector, where we use a lot of different dashboards.
Jose Luis: I agree with Carlos, but I must point out that QlikView is still in operation. As you know, I worked with you at Orange, and I can assure you that Qlik is alive and well. Recently, I have been using Quarto to create reports for my customers and business owners. I have found Quarto to be an effective tool for communicating my results. I have used Quarto to create slides, reports, and interactive documents. I am very pleased with Quarto’s ability to help me share my analysis. I may also use “conflr” library to create analyses in R markdown and publish them directly to Confluence. This would allow me to create an analysis and immediately publish the documentation, which would be a great time-saver.
Pedro:You inquired about what we miss or would like to see in the R environment. I am not familiar with the web services provided by Posit or RStudio. However, I would like to see something similar to Google Colab. Google Colab for universities is a marvel. You have access to really powerful machines and a lot of RAM for free. It is a freemium service, but you can still use it. I am not aware of an equivalent in the R sector.
Carlos: Posit Cloud’s free tier provides a very small account with only one gigabyte of storage. This is insufficient for many users, especially those who need to use GPUs for machine learning or data science. Posit is not as powerful as the Google Colab Platform. It would be beneficial for Posit to offer a GPU-enabled tier for users who need more powerful hardware.
Carlos: The use of GPUs in deep learning is a topic that is open to debate. Python dominates the field, and has a wide range of packages and libraries available for deep learning, such as PyTorch. R has an older library for deep learning, but it is not as widely used as Python. Many of the latest developments in deep learning, such as generative AI, are primarily done in Python. Therefore, for R to be used more widely for deep learning, it needs to have faster and more compatible libraries, as well as a more user-friendly interface.
Pedro: The development of R is progressing at a rapid pace, perhaps too quickly for the requirements of R libraries. This is because the libraries are constantly changing, with major changes occurring every six months. As a result, users must keep a close eye on the third number in the library versions to ensure that they are using the latest and greatest version. This can be a bit messy, but it is also exciting to see the language evolving so quickly.
Jose Luis: I used iGraph and visNetwork to conduct social network analysis on mobile phones in order to communicate my findings. I also used Spark to perform social network analysis (library Graphframes), but I presented the results to my customers using R. It was a large project.
Any upcoming projects that you might want to discuss?
Jose Luis: I have an upcoming talk planned in which I will discuss the use of R in production. You have seen API, Docker, and other technologies. Perhaps sharing Azure in AWS and Google Cloud. I believe I attempted to teach too much. However, it may be beneficial to teach others how to use R in production. I would start with simple automation, such as using the cronR R Package and taskscheduleR in Windows. The next step would be to use an API. Then Docker. Then Kubernetes. Finally, publish and deploy on a cloud platform.
Carlos:In my case, I calculate projections for different types of time series on a monthly basis. This can involve up to 3,000 to 4,000 different time series, which are calculated automatically and in a distributed manner. These projections are already in production, and I use them to track different KPIs for different economic sectors in Spain. This is of great importance to my industry, as it allows us to identify which sectors are growing and which are declining so that we can invest in the right areas. This is a live system that is of great value to us.
Francisco: In my case, I am working on the first level of the problem that Jose Luis mentioned. My objective is to predict which customers are likely to default on their payments. After a customer makes a purchase online, they have to pay the first installment. My goal is to predict the probability that the second installment will not be paid.
I have developed a fully automated process that collects all the data from a SAS database. The process extracts the data, loads it into a model, applies the model, and then prepares the data for another application. The other application uses a model to prioritize customers who are likely to pay, and then selects a subset of customers to send SMS or email reminders to.
This process is fully automated and runs every day between 10am and 6pm. It takes only five minutes to complete.
Pedro: In my case, I will be presenting a paper on psychometrics at the Barcelona Congress. Psychometrics is a niche area of statistics that focuses on psychological measurement. R is the only software that offers advanced psychometrics models. I will be using one of the oldest but still classical models. I have prepared a table of the current status of psychometrics models in R, which I may include in my presentation. This is because R is the only option for psychometricians nowadays. The big suites do not offer this software. This is another example of why R is the only way to go for these niche areas.
How do I Join?
R Consortium’s R User Group and Small Conference Support Program (RUGS) provides grants to help R groups organize, share information, and support each other worldwide. We have given grants over the past four years, encompassing over 65,000 members in 35 countries. We would like to include you! Cash grants and meetup.com accounts are awarded based on the intended use of the funds and the amount of money available to distribute.